In Image processing, we often use Signal to Noise Ratio (SNR) and Peak Signal to Noise Ratio (PSNR) for quality measurement.
Discrete signal power is defined as Let us now interpret this result. This is the ratio of the power of signal to the power of noise. Power is in some sense the squared norm of your signal. It shows how much squared deviation you have from zero on average. You should also note that we can extend this notion to images by simply summing twice of rows and columns of your image vector, or simply stretching your entire image into a single vector of pixels and apply the one-dimensional definition. You can see that no spacial information is encoded into the definition of power. Now let's look at peak signal to noise ratio. This definition is If you stare at this for long enough you will realize that this definition is really the same as that of Now, why does this definition make sense? It makes sense because the case of SNR we're looking at how strong the signal is to how strong the noise is. We assume that there are no special circumstances. In fact, this definition is adapted directly from the physical definition of electrical power. In case of PSNR, we're interested in signal peak because we can be interested in things like the bandwidth of the signal, or number of bits we need to represent it. This is much more content-specific than pure SNR and can find many reasonable applications, image compression being on of them. Here we're saying that what matters is how well high-intensity regions of the image come through the noise, and we're paying much less attention how we're performing under low intensity. |
Digital Image Processing
Sunday, November 17, 2013
Difference between SNR and PSNR
What is Time domain and Frequency domain?
Time/Frequency are interrelated parameter of a signal and both representations are two views of a same signal. Most of the time in practice, the signal measuring, is a
function of time. That is TIME-DOMAIN. In other words, when we plot the signal one of the axes is
time (independent variable), and the other (dependent variable) is usually the
amplitude. When we plot time-domain signals, we obtain a time-amplitude
representation of the signal.
This representation is not always the best
representation of the signal for most signal processing related applications.
In many cases, the most distinguished information is hidden in the frequency
content of the signal. The frequency SPECTRUM of a signal is basically
the frequency components (spectral components) of that signal. The frequency
spectrum of a signal shows what frequencies exist in the signal. The below represents a signal in time domain and frequency domain.
Demo :
In the above demo :
Red Color --- TIME DOMAIN SIGNAL
Blue Color --- FREQUENCY DOMAIN SIGNAL
MATLAB Code:
clear all; clc; close all; f=50; A=5; Fs=f*100; Ts=1/Fs; t=0:Ts:10/f; x=A*sin(2*pi*f*t); x1=A*sin(2*pi*(f+50)*t); x2=A*sin(2*pi*(f+250)*t); x=x+x1+x2; % Creating Hybrid signal which will have more than 1 frequency. plot(x) F=fft(x); figure N=Fs/length(F); baxis=(1:N:N*(length(x)/2-1)); plot(baxis,real(F(1:length(F)/2)))
Ideal Low Pass Filter Concept in MATLAB
%IDEAL LOW-PASS FILTER
%Part 1
f=imread(X); % reading an image X
P=input('Enter the cut-off frequency'); % 10, 20, 40 or 50.
[M,N]=size(f); % Saving the the rows of X in M and columns in N
F=fft2(double(f)); % Taking Fourier transform to the input image
%Part 2 % Finding the distance matrix D which is required to create the filter mask
u=0:(M-1);
v=0:(N-1);
idx=find(u>M/2);
u(idx)=u(idx)-M;
idy=find(v>N/2);
v(idy)=v(idy)-N;
[V,U]=meshgrid(v,u);
D=sqrt(U.^2+V.^2);
%Part 3
H=double(D<=P); % Comparing with the cut-off frequency to create filter mask
G=H.*F; % Multiplying the Fourier transformed image with H
g=real(ifft2(double(G))); % Inverse Fourier transform
imshow(f),figure,imshow(g,[ ]); % Displaying input and output image
end
Demo:
Reading an image:
Taking fourier transform of the above image, we get:Based on the cut-off freq (P) we design the filter function H , Here the cut-off frequency is nothing but radius of the white circle in the below image. The below image is usually referred as filter mask.
Performing filtering by using G=H.*F;% Multiplying the Fourier transformed
image with the filter mask H. Please note the convolution in time domain to equal to multiplication in frequency domain.
To the filtered output, we take inverse fourier transform for the above image and we get: This is the Ideal Low pass filtered image.
Wednesday, November 13, 2013
What is Digital Image Processing?
I will be using simple language to explain the concepts. To understand Digital Image Processing, let us see each word individually.
Image:
For standard definitions about image you can refer to the wikipedia page : Image Definition . If you have any difficulty in understanding that definitions, here is my definition for the image.
"Image" is the visual representation of information. That's it. Short and simple. If you are a person who isn't sure what the word "information" means, read further. "Information" is description of something or anything. To understand what the "visual representation" means here, read the below example.
I already told that "Information" is description of something. For example, my physical description is "Male, Young, Average height, Short hair, and Casually dressed". Here I have described my physical appearance in text, this is said to be representing an information through text.
The same information if I had communicated to someone through my speech, it is said to be representing an information through audio.
Similarly, the same information can be represented visually if I take a picture of myself and post it here.
The above image also conveys the same information i.e) "Male, Young, Average height, Short hair, and Casually dressed" but it is represented visually. So we have now learned what is an image in an unconventional way.
Digital:
A digital image is a numeric representation of a two-dimensional image. It will have pixel values are numerical.
What is pixel ?
Pixel is a smallest sample in an image. To understand what is pixel, you must know how the images are captured digitally(without using film rolls). Watch the below video:
Youtube Link: How to capture image digitally
I believe you have seen the above mentioned video. The smallest unit in the CMOS sensor is said to be the picture element. The term PIXEL is derived from this "PICture ELement". When the light reflected from the object that has to be captured hits this picture element in the CMOS sensor, the light energy is converted into electrical signals then this electrical signal converted into digital data (numerical value) using Analog to Digital Converter while saving to the memory card. So the image is now captured digitally.
Processing:
Now that we have captured an image digitally. We to do perform some operation on the captured image to improve its perceived quality (It is said to be Image enhancement), reduce the size for storage and transmission (Image Compression) and etc.
Here is the block diagram for different processes that are done for digital images:
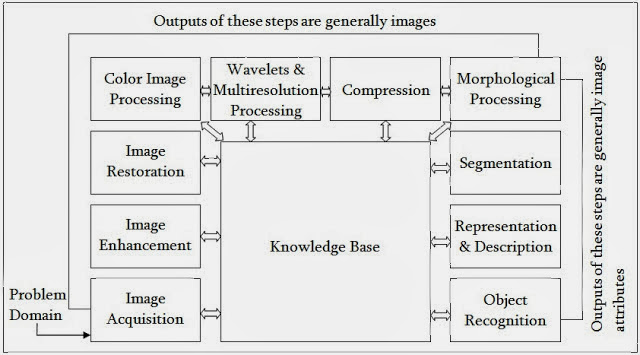
I hope this give you the basic understanding of Digital Image Processing.
Thirukural
Quote:
In sandy soil, when deep you delve, you reach the springs below;Explanation:
The more you learn, the freer streams of wisdom flow.
Water will flow from a well in the sand in proportion to the depth to which it is dug, and knowledge will flow from a man in proportion to his learning.Quote:
So learn that you may full and faultless learning gain,Explanation:
Then in obedience meet to lessons learnt remain.
Let a man learn thoroughly whatever he may learn, and let his conduct be worthy of his learning.
Subscribe to:
Posts (Atom)